Marketing Attribution Techniques: MMM, MTA, and Algorithmic Attribution
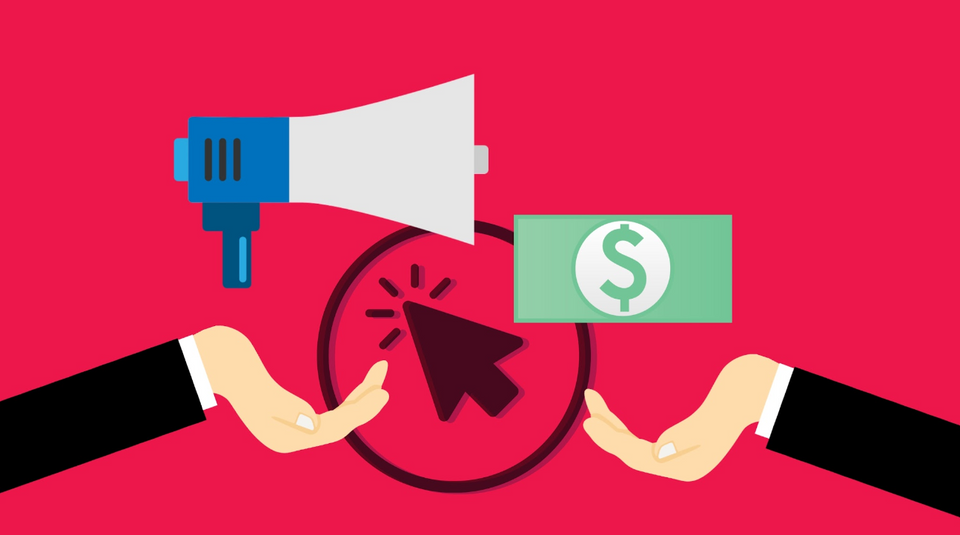
In today's digitally driven world, marketers face the challenge of accurately attributing business outcomes, like sales or leads, to a multitude of marketing channels. Among the numerous strategies used to tackle this issue are Marketing Mix Modeling (MMM), Multi-Touch Attribution (MTA), and Algorithmic attribution such as Regression-Based Attribution.
This article will dissect these techniques, provide concrete examples of their use, and demonstrate how they can be harmonized for a comprehensive marketing measurement approach.
Marketing Mix Modeling (MMM) - MMM is a top-down technique that uses historical, aggregated data to evaluate the impact of various marketing inputs on sales or market share.
Example Input Table for MMM: Here's a simplified example of input data for MMM. The data is usually aggregated at a weekly or monthly level.
Solution Design:
We can use regression analysis to model the impact of TV and Email spends, as well as macroeconomic factors, on Total Sales. Here's a simplified version of what the regression equation might look like:
Total Sales = b0 + b1*TV Spend + b2*Email Spend + b3*GDP + b4*UnemploymentRate
Output Table:
The output of MMM would typically be a set of coefficients (b0, b1, b2, b3, b4) that quantify the impact of each variable on sales.
Multi-Touch Attribution (MTA) - I have written extensively about MTA in this article. MTA is a bottom-up approach, it works at a user-event level and tries to assign credit to different touch points along the customer's journey.
Example Input Table for MTA: Here's a simplified example of input data for MTA:
Solution Design:
A simple solution would assign more credit to the first and last touchpoint and less to the middle ones, commonly known as the U-shaped attribution model.
Output Table:
The output will be an attribution table showing how much credit each touchpoint receives for each conversion:
Algorithmic Attribution (Regression)
Algorithmic Attribution, for example Regression based, uses regression analysis to model the relationship between marketing inputs and sales, similar to MMM, but potentially on a more granular level. The input, solution, and output for RBA would be similar to MMM. The primary difference is the level of data granularity. For instance, we might use daily data instead of weekly data, which might lead to different coefficients.
Combining MMM, MTA, and Algorithmic
For a comprehensive view, we can use a combined approach. MMM can provide a broad understanding of channel effectiveness. Algorithmic, with its flexible data granularity, can reveal insights at a more detailed level. MTA, on the other hand, helps understand user-level interactions.
Conclusion - Each technique—MMM, MTA, RBA—offers unique insights, and a blend of these methods can provide a well-rounded understanding of marketing effectiveness. This combined view can help marketers fine-tune both their strategic and tactical decisions, driving a more effective allocation of marketing resources. As always, it's critical to validate models against historical data to ensure their accuracy and applicability in your specific context.
Member discussion