Marketing Science: Using Confusion Matrix and Cost Function to Optimize Acquisition Marketing ROAS
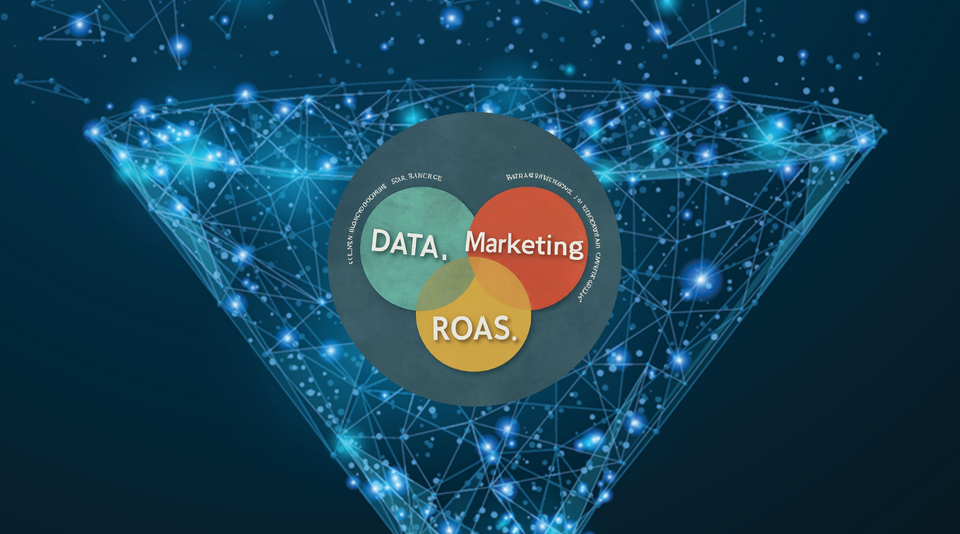
In my Day to Day Job, most of my conversations are around Data Driven Marketing and leveraging the power of Advanced Analytics in the field of marketing. One of the most talked about KPI’s in the world of Marketing is R.O.A.S as well as Predicted ROAS
I firmly believe that, to succeed in today's competitive landscape, marketers need to go beyond vanity metrics like clicks and conversions.
You need to know how much revenue each campaign is actually generating, and where you might be wasting money.
That's where the confusion matrix and cost function becomes your best friend. These tools give you a granular look at campaign performance, revealing hidden opportunities to refine your targeting, messaging, and overall strategy.
Confusion Matrix ?
Folks who have leveraged any of the ML techniques to solve a classification problem (Predicting labeled output within Supervised Learning), must have come across Confusion matrix.
A confusion matrix is a table that summarizes the performance of a classification model, like the one we use to predict potential customer conversions in acquisition campaigns.
It breaks down predictions into four categories:
- True Positives (TP): Customers we predicted would convert, and they did.
- True Negatives (TN): Customers we predicted would not convert, and they didn't.
- False Positives (FP): Customers we predicted would convert, but they didn't (also known as Type I error).
- False Negatives (FN): Customers we predicted would not convert, but they did (also known as Type II error).
Here's how a confusion matrix might look:
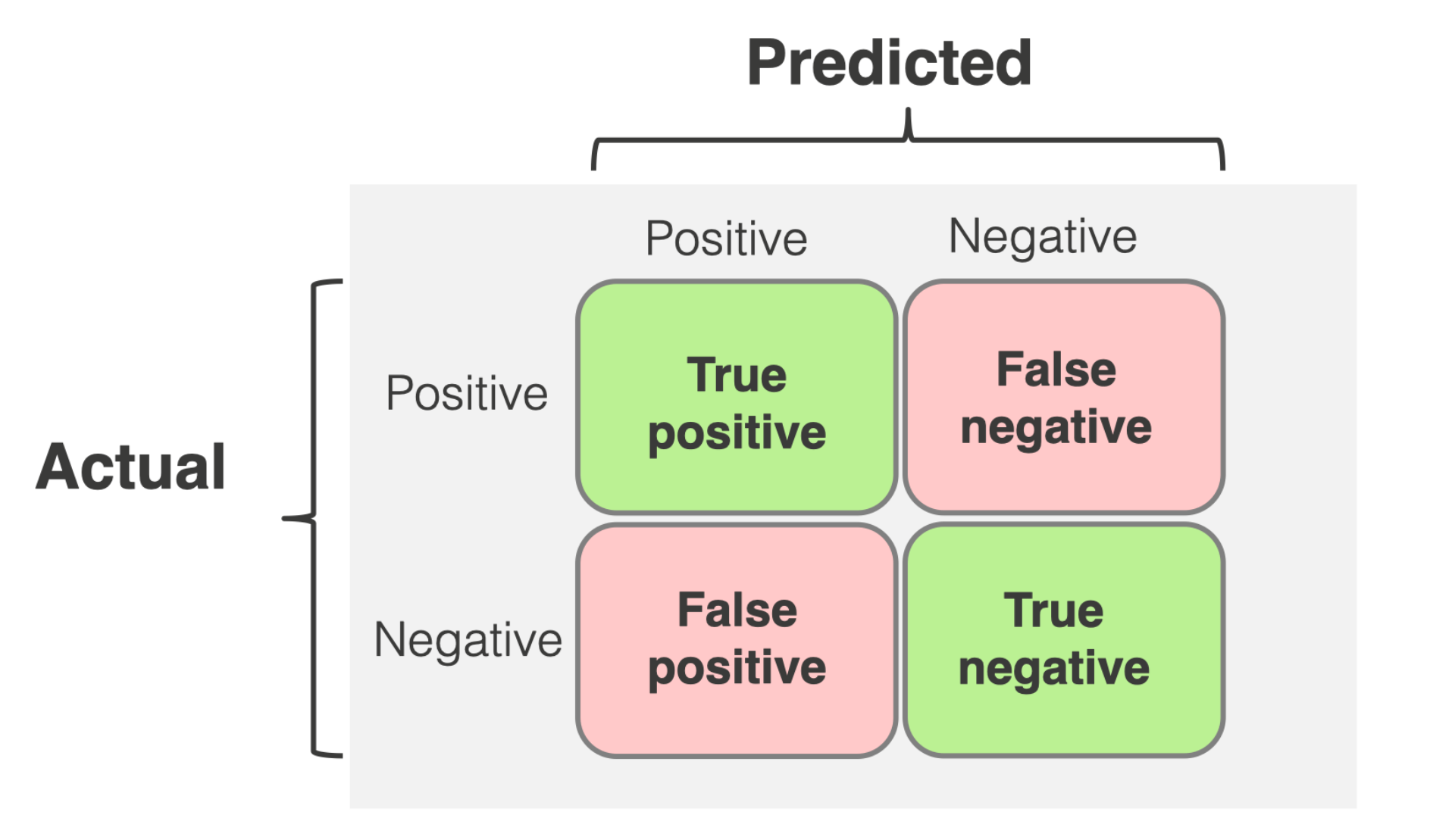
Let’s give more color to this by taking a simple business Example
Let's say we run a campaign targeting potential customers for a new subscription box. Analyzing the confusion matrix, we find a high number of False Positives. This means we spent money targeting customers who ultimately didn't subscribe. By analyzing this segment, we might discover they share certain characteristics, allowing us to refine targeting and avoid similar audiences in the future.
Measuring the Price of Mistakes - The Cost Function
You can further get more value out of your Confusion matrix by using The cost function
This assigns a cost to each type of error in the confusion matrix. This helps us understand the financial implications of misclassifications and prioritize which errors to minimize.
For example:
- Cost of FP (CFP): This could be the marketing spend wasted on targeting customers who didn't convert.
- Cost of FN (CFN): This could be the potential revenue lost from missing out on customers who were actually interested.
(Re)Calculating ROAS leveraging the Cost Function from the Confusion Matrix:
By combining the confusion matrix with the cost function, we can predict a more accurate ROAS. Here's a simplified formula:
ROAS = (Revenue from TP - Cost of FP) / Total Marketing Spend
Business Example: Continuing with our subscription box example, let's say the subscription cost is $50, CFP is $10, and CFN is $40. If we have 100 TP and 50 FP, the ROAS calculation would be:
ROAS = (100 $50 - 50 $10) / Total Marketing Spend
This gives us a clearer picture of the actual return on our investment, taking into account the cost of mis-classifications.
Optimizing Acquisition Campaigns with Data
By using the confusion matrix and cost function, we gain valuable insights into our acquisition campaigns, allowing us to:
- Refine Targeting: Identify and avoid segments with high FP rates.
- Adjust Campaign Strategies: Prioritize efforts towards minimizing costly errors (e.g., FN for high-value customers).
- Allocate Budget Effectively: Invest in channels and strategies that yield the highest ROAS.
You can go even further by leveraging another advanced analytics technique, which is Customer Segmentation. With this you could analyze the confusion matrix for different customer segments to tailor strategies accordingly.
By leveraging the power of data science, we can move beyond surface-level metrics and gain a true understanding of campaign performance, ultimately driving better ROAS and acquisition success.
Please let me know your thoughts on this!
Member discussion