Part 3: Unlocking Customer Insights: Leveraging GA4 Data in BigQuery for Enhanced Customer Segmentation
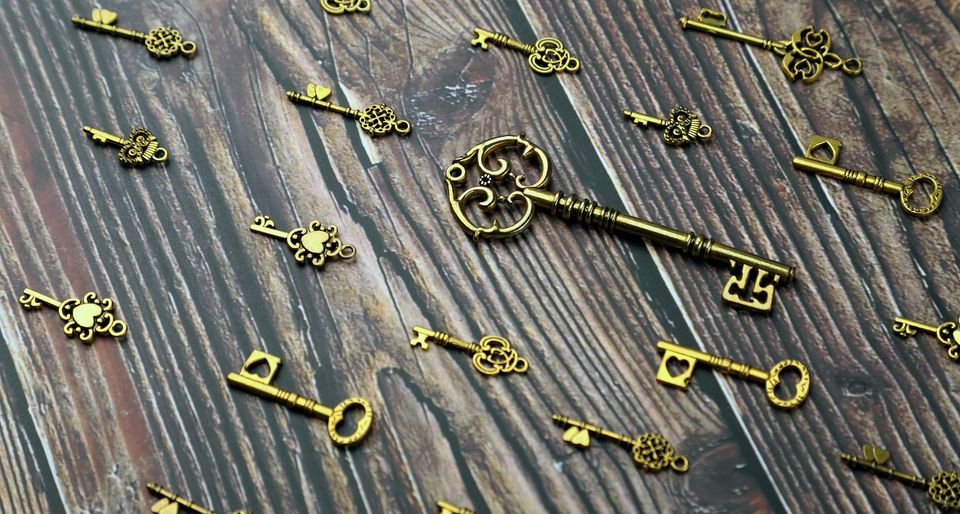
Welcome to Part 3 of our blog series on customer segmentation using GA4 data and BigQuery Vertex AI. In this final installment, I will guide you through the exciting process of training and evaluating ML models using BigQuery ML's powerful K-Means algorithm. By leveraging the capabilities of BigQuery ML, we can identify meaningful clusters within our data, unlocking valuable insights that will drive data-driven marketing strategies. Join me as we delve into the training and evaluation of ML models, empowering you to make informed decisions and tailor your marketing approaches to specific audience segments. Get ready to unlock the true potential of customer segmentation as we embark on this final chapter of our series.
Train using BigQuery ML K-Means Algorithm
Now, it's time to harness the immense power of the BigQuery environment as we directly leverage the BQML K-Means algorithm to achieve our objectives. With this robust tool at our disposal, we can unlock the potential of our data and extract valuable insights by applying the K-Means clustering algorithm. Let's dive in and explore the capabilities of BigQuery ML to accomplish our task efficiently and effectively.
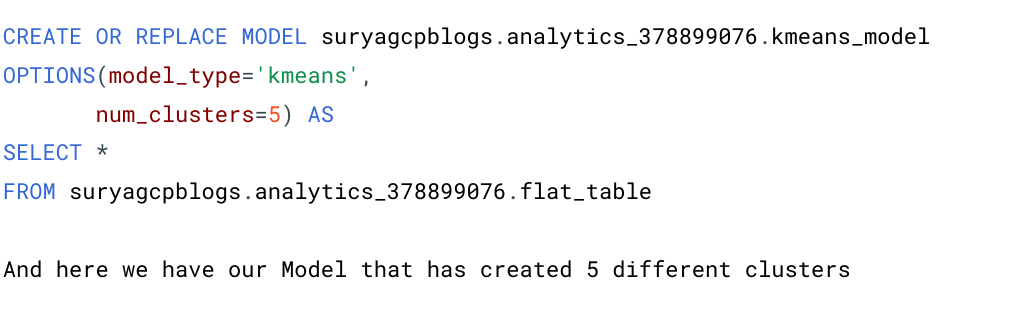
Upon completing the execution of the process, we have accomplished the generation of a model that successfully yields five distinct clusters. These clusters emerge as invaluable segments that can be utilized for in-depth analysis and the formulation of data-driven marketing strategies. With the formation of these clusters, we gain a deeper understanding of our data, allowing us to tailor our marketing efforts to specific audience segments, enhance personalization, and optimize campaign performance. These clusters provide a solid foundation for targeted marketing initiatives, facilitating more precise and effective engagement with our audience.
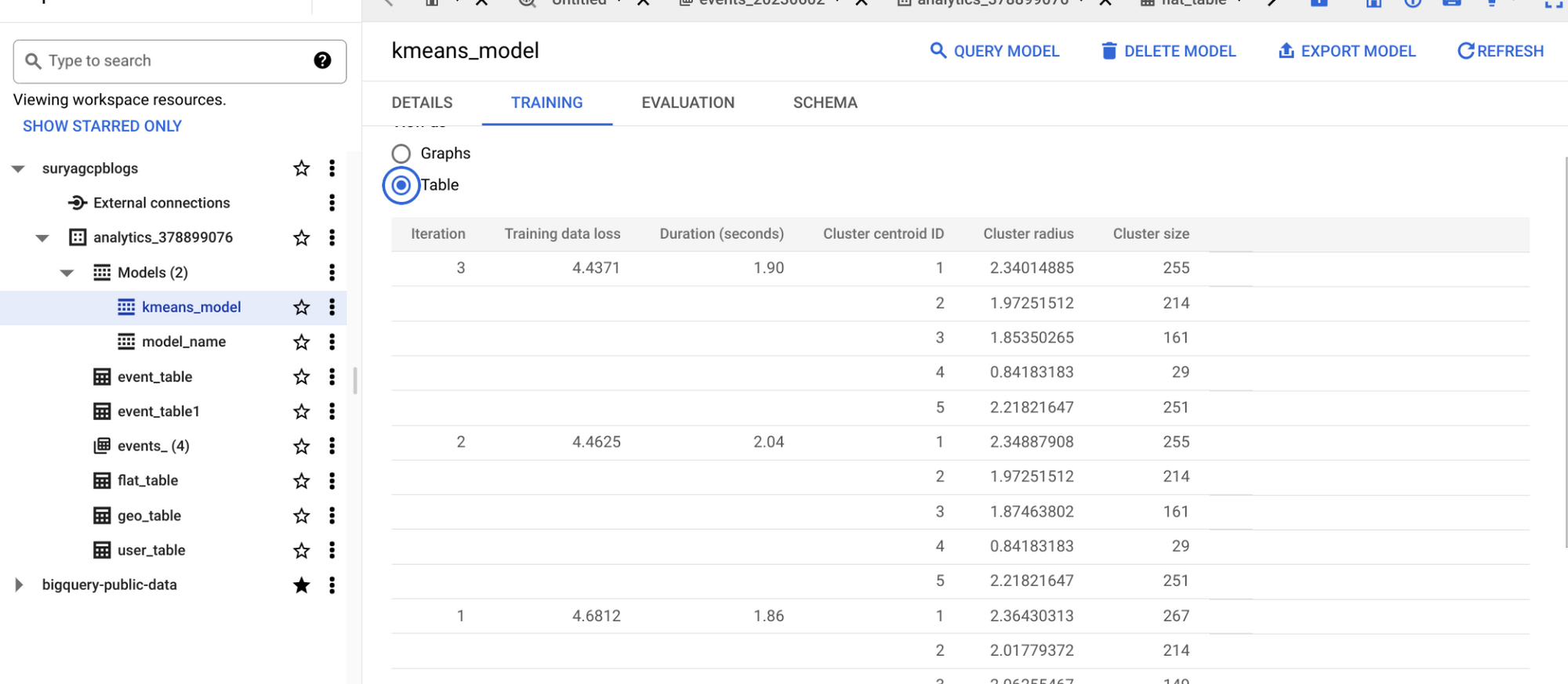
Evaluate BigQuery ML models
In the Evaluation tab, you will find visual representations of the clusters identified by the k-means model. Specifically, under the Numeric features section, bar graphs are presented, showcasing the top 10 significant numeric feature values associated with each centroid. You have the flexibility to choose the features you want to visualize by selecting them from the drop-down menu. This visualization provides valuable insights into the composition and characteristics of each cluster.
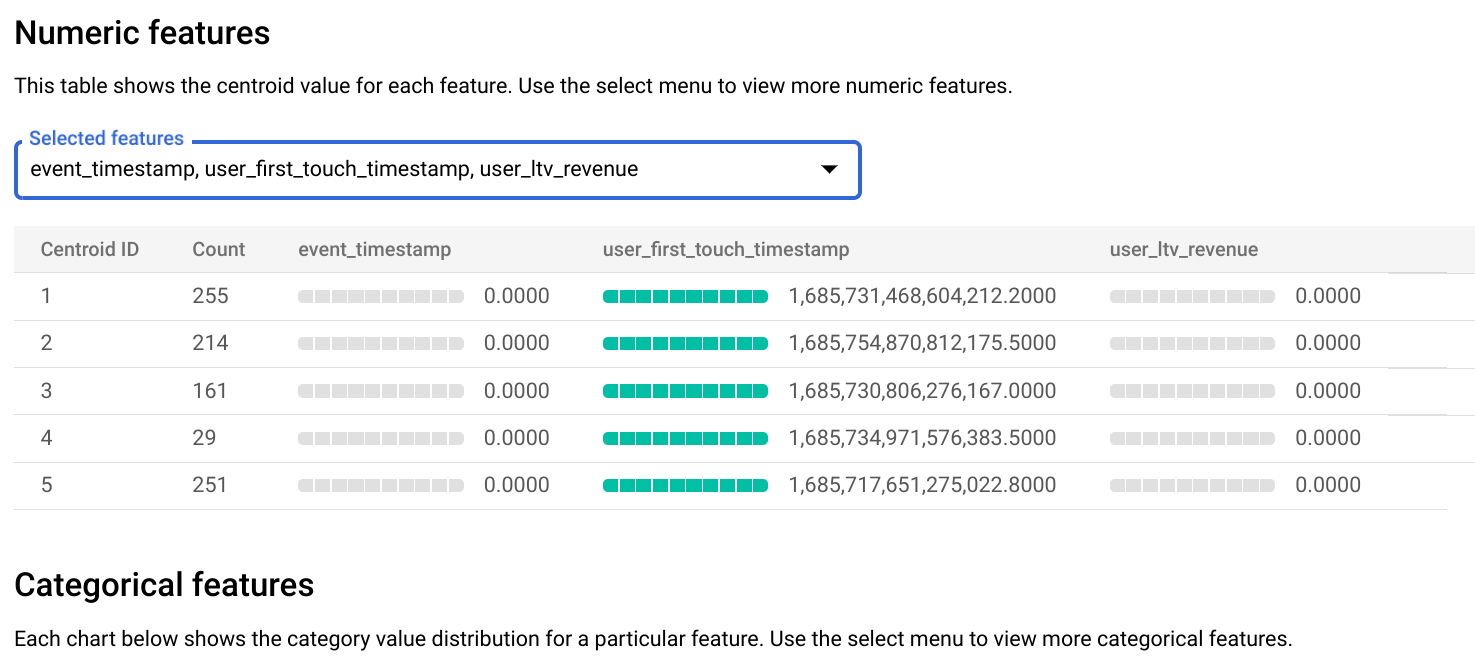
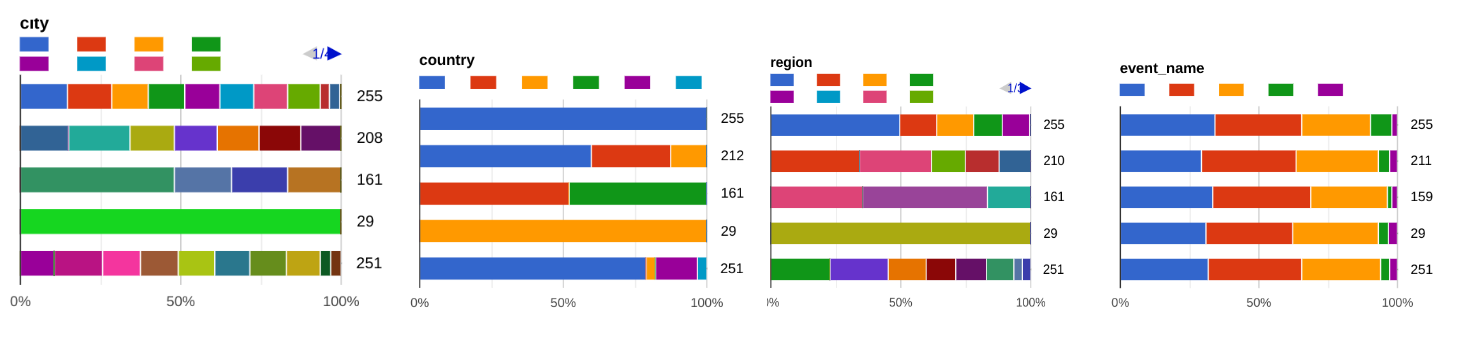
Through the appending of these cluster IDs, we can efficiently establish audience segments characterized by Centroid_IDs. These segments become readily accessible and invaluable for integration into our data-driven campaigns. This integration enables us to customize and refine our marketing endeavors, directing them towards specific clusters. The outcome is a more focused and personalized approach, leading to heightened engagement, improved campaign effectiveness, and ultimately, enhanced results. With the ability to target specific audience segments, we can tailor our messages, offers, and experiences to resonate more effectively with each cluster, forging stronger connections and driving better outcomes.
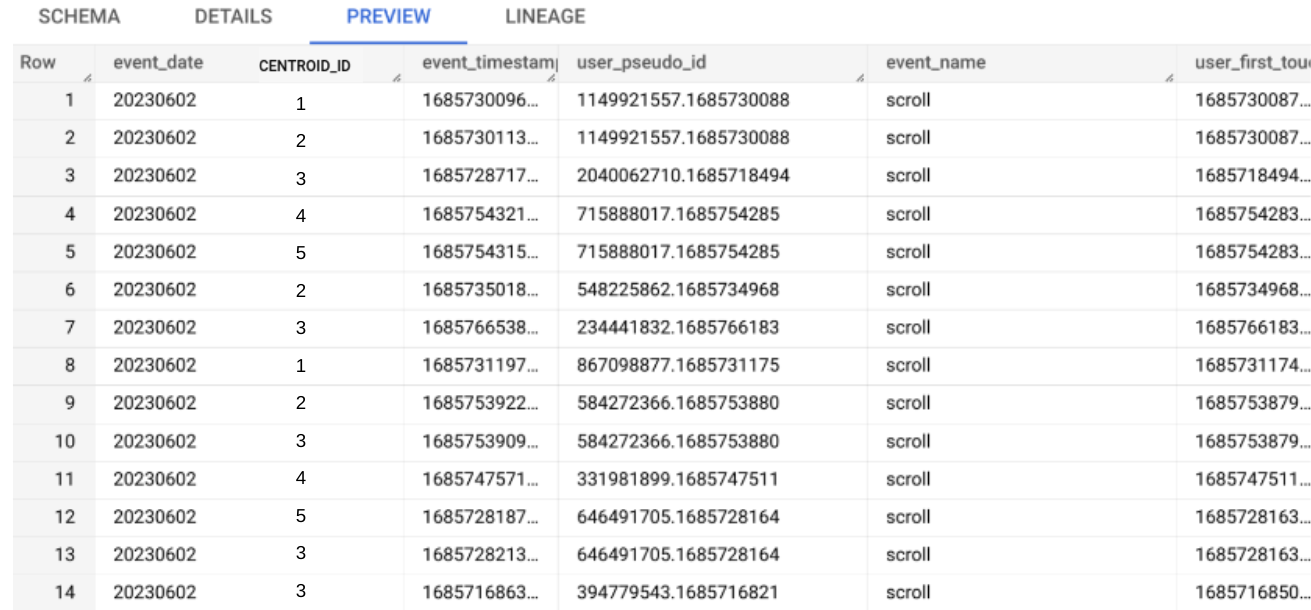
As an example Cluster 1
Segment Name: The Loyal Customers
Persona: The Loyal Customers segment represents individuals who have displayed long-standing loyalty to your brand. They are typically older and possess a higher income. These customers value and seek out quality products and services that they can trust.
Recommended Marketing Approach: Engage this segment by sending a personalized email featuring a special offer or exclusive discount. This gesture demonstrates your appreciation for their loyalty and encourages them to maintain their ongoing patronage.
In this illustrative example, Cluster 1 signifies a segment comprising loyal customers with unique personas and preferences. By comprehending the intricacies of this cluster and adapting marketing strategies accordingly, we can nurture and fortify our relationship with these customers, fostering long-term loyalty and maximizing their lifetime value.
In conclusion, the outcome of this endeavor has yielded five distinct clusters derived from our comprehensive flat_table. These clusters can be seamlessly exported as audiences within Google Analytics 4 (GA4), thereby equipping us with a potent tool for harnessing the potential of these segments across diverse advertising platforms like Google Ads or Display & Video 360 (DV360).
By effectively leveraging these audience segments, we can optimize our advertising strategies, ensuring that the right messages reach the right audience at the right time. This personalized approach leads to enhanced campaign performance, ultimately yielding better results and outcomes. The realm of data-driven marketing now expands, offering boundless possibilities for precise targeting and improved campaign efficacy.
With these powerful insights and tools at our disposal, we are poised to unlock the full potential of data-driven marketing, achieving higher levels of engagement, conversion, and overall success in our advertising endeavors.
If you have any questions or want to have any discussion on this topic, please reach out to me at LinkedIn.
Member discussion